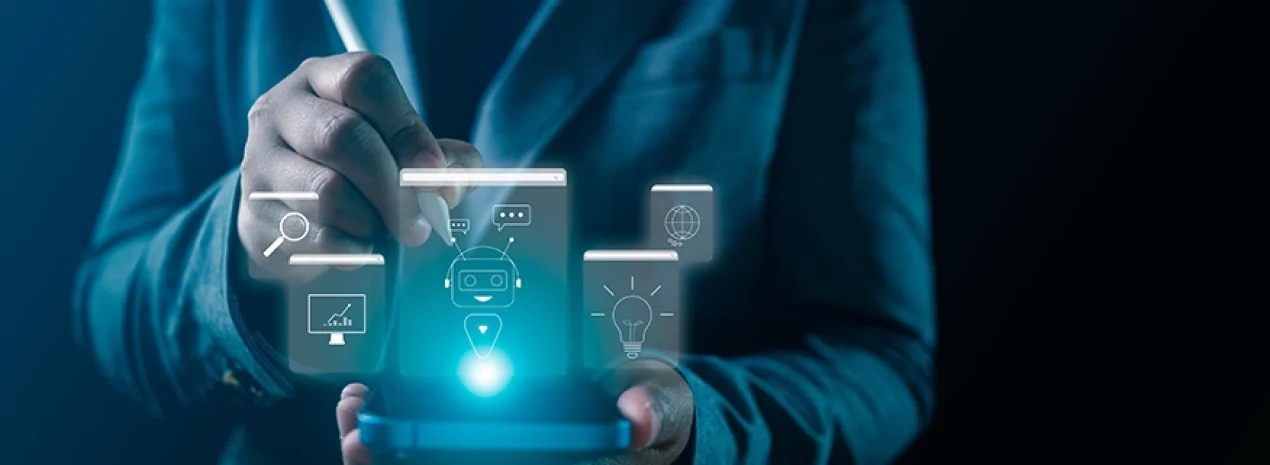
What is Data Discovery? Steps, Strategy & Best Practices Explained
Data discovery is a crucial process in today’s data-driven world. It involves exploring, classifying, and analyzing data from multiple sources to uncover patterns and generate actionable insights. This helps businesses make informed decisions, optimize processes, and identify opportunities for innovation and efficiency.
In this blog, we’ll dive into the concept, steps involved, strategies for effective data discovery, and best practices to ensure success.
What is Data Discovery?
It is the process of exploring, collecting, and analyzing data from various sources to detect trends, patterns, and anomalies. It allows organizations to navigate through large volumes of data and extract meaningful insights that can drive business decisions. Whether performed manually or through automated tools, the objective of data discovery is to transform raw data into valuable insights that enhance business intelligence.
Think of data discovery as a treasure hunt — it involves gathering and sifting through data to reveal insights that can improve decision-making, streamline operations, and drive innovation.
Why It Matters?
The importance spans across industries and sectors. It’s instrumental in helping businesses:
- Optimize operations: By highlighting inefficiencies and bottlenecks, data discovery enables businesses to streamline processes and enhance performance.
- Improve customer experiences: Analyzing customer behavior data helps businesses create personalized experiences and improve products and services.
- Drive product innovation: Data insights guide businesses in developing products or updates that meet customer expectations and market trends.
- Ensure compliance and security: By identifying sensitive data, businesses can meet regulatory requirements like GDPR or HIPAA and implement robust security practices.
Moreover, it fosters collaboration within organizations, making data accessible to everyone and promoting a data-driven culture.
Steps in the Data Discovery Process
It is typically a cyclical, iterative process involving the following six steps:
- Goal Setting: Begin by defining the objectives of the. What are you trying to achieve? Whether it’s uncovering customer behavior patterns or optimizing business operations, clear goals ensure that the data discovery process remains focused.
- Data Aggregation: Collect and consolidate data from various sources, including databases, spreadsheets, cloud storage, and social media platforms. Aggregating data provides a holistic view and allows for more comprehensive analysis.
- Data Preparation: Before analyzing the data, it’s important to clean and standardize it. This step involves removing duplicates, handling missing values, and ensuring data consistency. Clean data ensures more accurate and reliable insights.
- Visualization: Data visualization tools like charts, graphs, and heat maps play a critical role in the data discovery process. They help uncover patterns and trends that may not be apparent in raw data.
- Data Analysis: Analyze the prepared data to extract meaningful insights. This can be done through statistical methods or advanced analytics, depending on the complexity of the data and the goals of the analysis.
- Repeat the Process: Data discovery is not a one-time event. As new data becomes available, it’s important to continuously refine your analysis to ensure that the insights remain relevant and valuable.
Data Discovery Methods: Manual vs. Automated
There are two primary methods: manual and automated.
- Manual Data Discovery: Involves manually collecting, organizing, and analyzing data. While it offers greater control and customization, manual discovery is time-consuming and requires deep knowledge of data organization.
- Automated Data Discovery: Leverages AI and machine learning to automate data aggregation, cleaning, and analysis. Automated tools streamline the process and enable non-technical users to derive insights without extensive expertise in data management. These tools can detect outliers, unify data formats, and apply machine learning algorithms to accelerate insights.
Strategy for Effective Data Discovery
To ensure successful a process, businesses should adopt a clear strategy that aligns with their goals. Here are some strategic considerations:
- Define Clear Objectives: Establish specific, measurable goals that the data discovery process should achieve. Without clear objectives, it can lead to irrelevant or insignificant results.
- Choose the Right Tools: Whether manual or automated, selecting the appropriate tools is key to efficient process. Automated tools like Tableau or Power BI can accelerate insights and enable self-service discovery for business users.
- Collaborate Across Departments: Ensure that stakeholders from different departments are involved. Cross-functional collaboration enriches the analysis and ensures that the data insights are aligned with organizational objectives.
- Prioritize Data Governance: Implement robust data governance policies to ensure data accuracy, security, and compliance. This includes defining data ownership, enforcing access controls, and regularly auditing the data.
Best Practices for Data Discovery
By following best practices, businesses can optimize their efforts and derive the maximum value from their data. Here are key best practices to consider:
- Focus on Data Quality: Clean, accurate data is critical to the success of the process. Make sure to clean and standardize data during the preparation phase to ensure high-quality analysis.
- Leverage Data Governance: Establish strong data governance frameworks to safeguard sensitive data and ensure compliance with privacy regulations. This includes defining data ownership, applying security controls, and tracking data usage.
- Encourage a Data-Driven Culture: Empower employees at all levels to participate in the data discovery process. This democratizes access to insights and fosters a collaborative, data-driven decision-making environment.
- Use Visualization Tools: Visualization tools like Tableau or QlikSense make it easier to interpret complex data and quickly identify trends. These tools also facilitate the sharing of insights across teams.
- Automate When Possible: Automation speeds up the process, reduces errors, and enables real-time insights. Use AI-powered tools to handle repetitive tasks like data cleaning and formatting.
Conclusion
Data discovery is a powerful tool that helps businesses uncover hidden insights, drive innovation, and optimize operations. By adopting the right strategies, using the appropriate tools, and following best practices, organizations can unlock the full potential of their data and make informed, data-driven decisions.
FAQs
How does data discovery differ from traditional data analysis?
Data discovery is more exploratory and iterative, focusing on uncovering hidden patterns and trends in raw data across multiple sources, while traditional data analysis typically involves predefined hypotheses and structured reporting of known data sets.
Can small businesses benefit from data discovery, or is it only for large enterprises?
Absolutely! The tools are scalable, meaning small businesses can leverage them to uncover insights about customer behavior, operational inefficiencies, and growth opportunities without the need for extensive IT infrastructure.
How does data discovery enhance compliance with data privacy regulations like GDPR or HIPAA?
It helps identify sensitive data across an organization, ensuring that it is correctly classified, protected, and managed. This reduces the risk of non-compliance by enabling better visibility and control over where sensitive information is stored and how it is used.
What role does machine learning play in automated data discovery?
Machine learning enhances the process by automating processes like data cleaning, anomaly detection, and pattern recognition. It can learn from data patterns and improve its accuracy over time, enabling faster and more reliable insights.
How can organizations ensure the security of data during the discovery process?
Implementing strict access controls, encrypting sensitive data, and regularly auditing data access are key practices. Data governance frameworks should be integrated with the tools to ensure that only authorized personnel can access and manipulate sensitive information.
Latest
Blogs
Whitepapers
Monthly Threat Brief
Customer Success Stories