Home » AI at Work: Risks of AI-Driven Data Exposure
AI at Work: Risks of AI-Driven Data Exposure
Share on
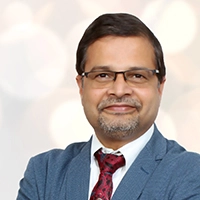
AI’s Growing Influence and the Unseen Risks
The widespread adoption of artificial intelligence in workplace environments is fundamentally reshaping business operations, driving both efficiency and complexity in enterprise data management. With over 80% of employees now leveraging AI-powered tools to optimize workflows, AI has rapidly transitioned from an emerging technology to an integral business function. However, as organizations embrace AI for automation, analytics, and decision-making, they also face a growing challenge: how enterprise data is stored, processed, and protected remains opaque.
Despite the industry’s rapid advancements, recent analyses of AI models have highlighted significant security gaps, exposing weaknesses in prompt filtering, data retention policies, and information exposure risks. Some AI models have been found susceptible to adversarial attacks, failing to block harmful, misleading, or policy-violating queries. These vulnerabilities raise critical concerns for enterprises entrusting sensitive corporate information to AI systems. Once business data enters an AI model, organizations often lack visibility into how it is handled, where it is stored, or whether it remains within system logs.
This challenge is exacerbated by the rise of Shadow AI—the unsanctioned use of AI applications by employees without formal approval or security oversight. A survey by Salesforce revealed that over a quarter of workers are currently using generative AI at work, with more than half doing so without their employer’s formal approval. AI systems that interact with sensitive data—such as customer records, financial reports, or proprietary algorithms—may inadvertently expose classified information to external servers, creating vulnerabilities that cybercriminals or unauthorized third parties could potentially exploit.
Incidents such as Samsung’s accidental exposure of confidential source code in ChatGPT illustrate the potential risks of AI-driven data leaks. More concerning is the potential for AI systems to absorb sensitive data into training models, even in cases where vendors claim that inputs are not permanently stored. As regulatory bodies worldwide refine privacy mandates such as GDPR, CPRA, and PCI DSS, organizations must evaluate the full risk landscape of AI tools before allowing them to process enterprise data.
The Privacy Challenge: Third-Party AI Monitoring and Enterprise Exposure
Recognizing these risks, some organizations have adopted AI monitoring tools to track and regulate how employees interact with AI systems. While well-intentioned, these solutions often introduce additional layers of risk. AI monitoring platforms typically log user queries, archive AI-generated responses, and produce detailed reports on AI engagement trends. However, these logs may store sensitive corporate data longer than necessary, inadvertently becoming a secondary repository of proprietary information.
A core question remains: Who has access to these AI logs? How long are they retained? Are they accessible by third parties? Without strict governance, AI monitoring tools could themselves become security liabilities, exposing organizations to insider threats, unauthorized data access, and compliance risks.
Additionally, threat actors have begun exploiting AI-driven vulnerabilities to extract corporate intelligence, manipulate authentication processes, and launch automated cyberattacks. The weaponization of AI for deepfake-based fraud is already well documented—in one instance, cybercriminals used AI-generated video calls to impersonate corporate executives, successfully manipulating financial transactions worth $25 million. The next frontier of cyber threats will likely involve leveraging AI models to craft highly personalized, context-aware phishing campaigns, bypassing traditional security filters and authentication measures.
As these risks evolve, CISOs and security leaders must ask themselves: Are we securing AI, or is AI exposing us? Organizations must establish comprehensive AI risk management strategies that account for third-party monitoring vulnerabilities, adversarial manipulation risks, and regulatory compliance gaps before they escalate into full-scale security incidents.
Building a Resilient AI Governance Framework
As AI adoption accelerates, businesses must ensure that AI-driven data interactions are governed effectively to mitigate security and privacy risks. AI tools must align with corporate security policies, compliance mandates, and risk tolerance thresholds to prevent unauthorized data access, model misuse, and regulatory violations. Without structured governance, organizations risk data exposure, compliance failures, and reputational damage. A resilient AI governance framework integrates the following points and approaches:
- Structured AI Governance Approach
- AI systems should be governed under a defined security framework that aligns with compliance mandates.
- Organizations must establish clear policies and controls to prevent unauthorized AI interactions.
- Zero Trust Security for AI
- AI tools should be treated as untrusted by default and granted only minimum necessary access.
- Segment AI workflows to restrict access to mission-critical datasets, reducing exposure risks.
- Robust Data Classification & Access Control
- Define data classification frameworks to regulate AI access to customer PII, financial models, and proprietary data.
- Enforce role-based access controls (RBAC) to ensure privileged access is strictly monitored and regulated.
- Vendor Due Diligence & AI Security Assessments
- AI security assessments should evaluate:
- Vendor disclosure policies on data retention
- Encryption practices and security controls
- Jurisdictional compliance and regulatory transparency
- Enterprises must ensure clear vendor policies on AI data storage, model training, and data deletion mechanisms.
- AI security assessments should evaluate:
- AI Literacy & Security Awareness Initiatives
- Train employees to recognize AI privacy risks and assess AI-generated outputs for accuracy.
- Educate staff on responsible AI usage, corporate security policies, and ethical AI considerations.
- Ensure awareness of emerging AI-driven cyber threats to foster a culture of secure and ethical AI adoption.
- Proactive AI Risk Assessment & Continuous Monitoring
- AI governance requires ongoing security assessments and compliance audits.
- Organizations must integrate AI monitoring into their security frameworks to prevent data misuse and regulatory violations.
Neglecting AI security governance today will lead to increased privacy risks, regulatory scrutiny, and potential reputational damage. Businesses must embed AI security governance into their foundational security strategies, ensuring AI-driven innovation is balanced with responsible data stewardship.
How SISA Supports Data Governance
As organizations navigate the evolving AI security landscape, SISA provides expert-driven solutions to help businesses develop robust security frameworks, regulatory compliance strategies, and enterprise data governance models. Through advanced risk assessments and tailored security solutions, SISA empowers organizations to deploy technology responsibly while mitigating data privacy risks.
To learn more about how SISA enables businesses to secure digital environments while ensuring compliance with global privacy regulations, connect with our experts today.