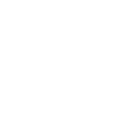
Recent months have sparked a growing interest in generative AI tools such as ChatGPT and Google Bard and the Large Language Models (LLMs) that underpin them. Amidst the hype, there is a darker side of AI that we need to rapidly address. One of the most significant areas of risk that generative AI systems present is in cybersecurity. Organizations’ rush to embrace generative AI may be ignoring the significant security threats that LLM-based technologies like ChatGPT pose, particularly in the open-source development space and across the software supply chain. The following section outlines the five most significant of these risks, providing insight into the challenges they pose for both data privacy and cybersecurity in today’s digital landscape:
Generative AI can mimic human-like behaviors and create realistic content, which can significantly enhance social engineering attacks. Malicious actors can utilize AI-powered chatbots to craft convincing and tailored messages to deceive individuals into disclosing sensitive information or clicking on malicious links. Video-based generative AI could super-charge deep fake attacks, can be used to bypass facial recognition security measures in an identity-based attack, or to impersonate company employees in spoofing attacks, while text-based generative AI tools such as ChatGPT can be used to create highly personalized emails to enable spear phishing at scale. Alternatively, an attacker could use an audio-generating model to create a fake audio clip of a CEO instructing employees to take a specific action. There have been several instances already reported where ChatGPT-based malicious campaigns are using different distribution channels to deliver malicious content, such as fake social media pages containing links to typosquatted or deceptive domains mimicking the real OpenAI website. Attackers have not limited themselves to fake social media pages but are also exploiting browser extensions with ChatGPT-themed attacks to steal session cookies or launch SEO poisoning attacks.
Attackers can train generative AI systems to generate sophisticated malware and automate vulnerabilities. Hackers can use AI-powered tools to generate polymorphic malware (malware that can dynamically change its code structure and appearance while retaining its core functionality). Traditionally, malware authors would have to manually modify their code to create new variants or use simple encryption techniques to obfuscate their malware. These capabilities will make it more difficult to detect and defend against malware. Bad actors are actively using tools such as WormGPT and FraudGPT – ChatGPT clones trained on malware-focused data to exploit vulnerabilities, launch business email compromise (BEC) attacks and create malware. This new ability poses a significant challenge for cybersecurity professionals as they grapple with increasingly intelligent and adaptive threats.
The generative AI models use and learn from the information and context that users input when creating a prompt. With most businesses still running pilots or building use cases without sufficient controls to check for data sharing, users may be sharing proprietary or confidential information with the AI chatbot. Research done by Cyberhaven, a data security company, found that 11% of data employees paste into ChatGPT is confidential. Such uncontrolled use of generative AI tools may lead employees to unintentionally share the company’s intellectual property, sensitive strategic information, and customer data with chatbots, elevating the risk of data breaches and identity theft. A data leak incident reported earlier this year exploited a bug in ChatGPT’s source code resulting in a breach of sensitive data, where unauthorized actors were able to view users’ chat history due to a vulnerability in the Redis memory database used by OpenAI. The incident also exposed personal and payment data of approximately 1.2% of active ChatGPT Plus subscribers. Instances like this may lead to sensitive data being stored, accessed or misused by malicious actors to launch targeted ransomware or malware attacks, that could disrupt business operations.
Generative AI algorithms can be trained to detect and exploit vulnerabilities in security systems, evading traditional defenses such as signature-based detection and rule-based filters. This technique leverages the power of AI to streamline the process of discovering and exploiting vulnerabilities, enabling malicious actors to identify and exploit them at scale and minimizing the manual effort required by the attackers. By automating the process, attackers can rapidly target numerous systems or software instances, increasing their chances of successfully compromising a target. This puts organizations at risk of data breaches, unauthorized access, and other security incidents.
Adversaries may deliberately manipulate the training data fed to a generative AI model to introduce vulnerabilities, backdoors, or biases that can undermine the security, effectiveness, or ethical behavior of the model. For example, ethical hackers have discovered new prompt injection attack targeting users of ChatGPT that modifies chatbot answer and exfiltrates the user’s sensitive chat data to a malicious third-party. It can be optionally extended to affect all future answers and making injection persistent. Such data poisoning instances can be especially problematic as it corrupts the entire generative process. If a generative AI model is exposed to poisoned data during training, it can produce harmful, biased or misleading outputs, leading to significant consequences when used in real-world applications. These could include spread of misinformation, perpetuating stereotypes and discrimination and damaging reputation.
The rapid adoption of generative AI is changing the threat landscape tremendously. Cyber vulnerabilities are becoming democratized and ransomware attacks, phishing schemes and malware creation easier and more ubiquitous. With enterprise adoption of generative AI only expected to accelerate, it is imperative that businesses adopt a proactive and comprehensive approach to cybersecurity. The first step is an awareness that integrating generative AI comes with unique challenges and security concerns that may be different than anything organizations have encountered before, requiring new controls for governance. Embracing a “secure-by-design” approach by leveraging existing frameworks like the Secure AI Framework (SAIF) or MITRE ATLAS to incorporate security measures directly into their AI systems is also key. It’s also imperative that organizations monitor and log LLM interactions and regularly audit and review the AI system’s responses to detect potential security and privacy issues.
References:
To get daily updates on the emerging threats and critical vulnerabilities being exploited by threat actors, subscribe to SISA Daily Threat Watch – our daily actionable threat advisories.
Blogs
Whitepapers
Threat-a-Licious
Customer Success Stories
SISA is a global forensics-driven cybersecurity solutions company, trusted by leading organizations for securing their businesses with robust preventive, detective, and corrective cybersecurity solutions. Our problem-first, human-centric approach helps businesses strengthen their cybersecurity posture.
Industry recognition by CREST, CERT-In and PCI SSC serves as a testament to our skill, knowledge, and competence.
We apply the power of forensic intelligence and advanced technology to offer true security to 2,000+ customers in 40+ countries.